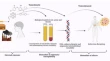
Overview
Journal of Environmental Health Science & Engineering presents timely research on all aspects of environmental health science, engineering and management.
Including Water pollution and treatment, Wastewater treatment and reuse, Air control, Soil remediation, Noise and radiation control, Environmental biotechnology and nanotechnology, Food safety and hygiene.
Journal of Environmental Health Science & Engineering is the official journal of the Iranian Association of Environmental Health (IAEH), published on behalf of Tehran University of Medical Sciences.
- Chair
-
- Alireza Mesdaghinia
- Editor-in-Chief
-
- Ramin Nabizadeh
- Impact factor
- 3.4 (2022)
- 5 year impact factor
- 3.5 (2022)
- Submission to first decision (median)
- 42 days
- Downloads
- 245,619 (2023)
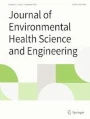
Latest articles
Journal information
- Electronic ISSN
- 2052-336X
- Abstracted and indexed in
-
- Astrophysics Data System (ADS)
- BIOSIS
- Baidu
- CAB Abstracts
- CLOCKSS
- CNKI
- CNPIEC
- Chemical Abstracts Service (CAS)
- DOAJ
- Dimensions
- EBSCO
- EMBiology
- Engineering Village – GEOBASE
- Gale
- Google Scholar
- INIS Atomindex
- Japanese Science and Technology Agency (JST)
- Naver
- Norwegian Register for Scientific Journals and Series
- OCLC WorldCat Discovery Service
- Portico
- ProQuest
- PubMedCentral
- SCImago
- SCOPUS
- Science Citation Index Expanded (SCIE)
- Semantic Scholar
- TD Net Discovery Service
- UGC-CARE List (India)
- Wanfang
- Copyright information